- Postdoctoral Position, National Institutes of Health, 1999-2003
- Ph.D., Yale, 1998
- B.S., Cornell University, 1985
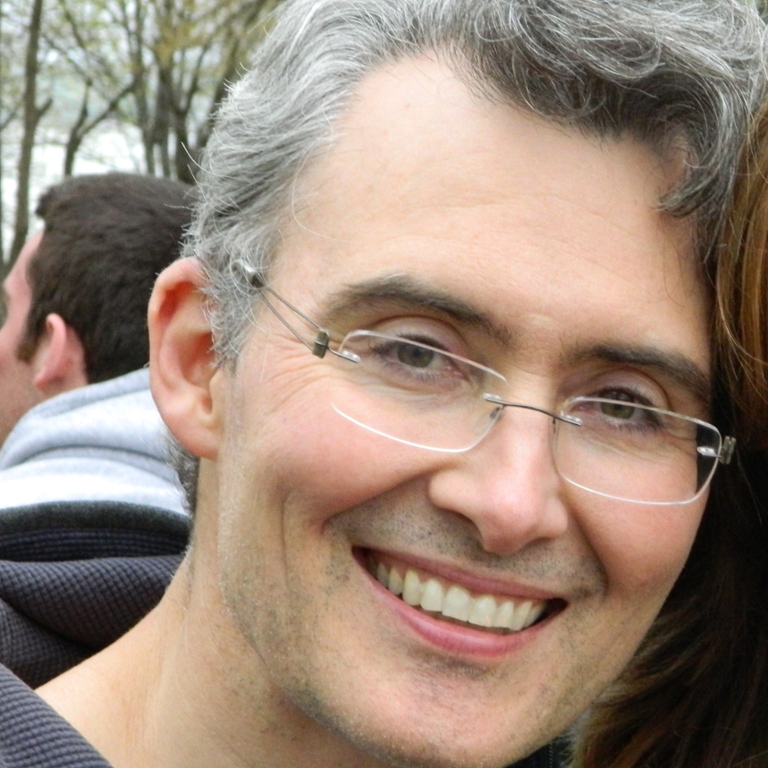
John M. Beggs
Professor, Physics
Professor, Physics
biophysics
The physical sciences have had great success in describing how complex phenomena can emerge from the collective interactions of many similar units. Waves, turbulence, phase transitions, and self-organization are all examples of this.
Although the brain is tremendously complex, it is composed of many units, neurons, which appear to be similar. This resemblance has led many researchers to borrow concepts from physics in an effort to explain neural function. Indeed, many models predict that neural networks should exhibit metastable states like those seen in frustrated magnetic materials, and should operate near a critical point like that seen in matter at a phase transition. While this body of theory has prospered, experiments to test it have been few.
Recent advances in technology, however, have allowed thousands of interconnected neurons to be grown on microfabricated arrays of many electrodes. These "brains in a dish" can be kept alive for weeks while their spontaneous electrical activity is recorded. The large data sets produced by these experiments have allowed many of the hypotheses inspired by statistical physics to be examined in real neural tissue.
Our results indicate that living neural networks do in fact organize themselves so that many metastable states exist. In addition, these networks appear to operate at the critical point, producing distributions of event sizes that can be described by a power law. This surprising correspondence between biological data and physical theory may actually serve a purpose for the networks. Simulations indicate that metastable states can be used to store information, and that the critical point optimizes information transmission while preserving network stability. Future research combining biological experiments and computer simulations will be directed toward understanding fundamental emergent properties of living neural networks and how these properties may contribute to neural function.
Nir Friedman, Shinya Ito, Braden Brinkman, Masanori Shimono, R. Lee DeVille, Karin Dahmen, John M. Beggs, and Tom C. Butler. Universal critical dynamics in high resolution neuronal avalanche data. Physical Review Letters 108, 208102 (2012). https://journals.aps.org/prl/abstract/10.1103/PhysRevLett.108.208102
John M. Beggs and Nick Timme. Being critical of criticality in the brain. Frontiers in Fractal Physiology. (2012). http://www.ncbi.nlm.nih.gov/pmc/articles/PMC3369250/pdf/fphys-03-00163.pdf
Nick Timme, Wesley Alford, Benjamin Flecker, John M. Beggs. Towards resolving the multivariate information controversy. (2012). https://arxiv.org/abs/1111.6857
Benjamin Flecker, Wesley Alford, John M. Beggs, Paul Williams, and Randall Beer. Partial information decomposition as a spatiotemporal filter. Chaos 21, 037104 (2011). https://aip.scitation.org/doi/10.1063/1.3638449
Shinya Ito, Mike Hansen, Randy Heiland, Andrew Lumsdaine, Alan Litke, and John M. Beggs. Extending transfer entropy improves identification of effective connectivity in a spiking cortical network model. PLoS ONE 6(11): e27431. (2011) doi:10.1371/journal.pone.0027431. https://journals.plos.org/plosone/article?id=10.1371/journal.pone.0027431
Jon P. Hobbs, Jodi L. Smith, and John M. Beggs. Aberrant neuronal avalanches in cortical tissue removed from juvenile epilepsy patients. Journal of Clinical Neurophysiology. 2010 Dec. 27(6):380-6.
Wei Chen, Jon P. Hobbs, Aonan Tang, and John M. Beggs. A few strong connections: Optimizing information retention in neuronal avalanches. BMC Neuroscience. 2010 Jan 6;11(1):3. Open access at: https://bmcneurosci.biomedcentral.com/articles/10.1186/1471-2202-11-3
Fang-Chin Yeh, Aonan Tang, , Jon P. Hobbs, Pawel Hottowy, Wladek Dabrowski, Alexander Sher, Alan Litke, and John M. Beggs. Maximum entropy approaches to living neural networks. Entropy, 12: 89-106, 2010. Open access at: https://bmcneurosci.biomedcentral.com/articles/10.1186/1471-2202-11-3
Hsu, D., Chen, W., Hsu, M. and Beggs, J.M. An open hypothesis: is epilepsy learned, and can it be unlearned? Epilepsy and Behavior, Jun 21, 2008. (Epub ahead of print).
Tang, A., Jackson, D., Hobbs, J., Chen, W., Prieto, A., Smith, J.L., Patel, H. Sher, A., Litke, A and Beggs, J.M. A maximum entropy model applied to spatial and temporal correlations from cortical networks in vitro. The Journal of Neuroscience, 28(2): 505-518, 2008.
Beggs, J.M. The criticality hypothesis: how local cortical networks might optimize information processing. Philos Transact A Math Phys Eng Sci. 366(1864):329-343, 2008. https://royalsocietypublishing.org/doi/full/10.1098/rsta.2007.2092
Beggs, J.M. How to build a critical mind. Nature Physics 3(12): 834-835, 2007.
Hsu, D., Tang, A., Hsu, M., and Beggs, J.M. Simple spontaneously active Hebbian learning model: Homeostasis of activity and connectivity, and consequences for learning and epileptogenesis. Physical Review E 76: 041909, 2007.
Beggs, J.M. Neuronal Avalanche. Scholarpedia, p.6423, 2007. http://www.scholarpedia.org/article/Neuronal_avalanche
Hsu, D. and Beggs, J.M. Neuronal avalanches and criticality: A dynamical model for homeostasis. Neurocomputing, 69:1134-1136, 2006.
Beggs, J.M. and Haldeman, C. Beggs and Haldeman Reply: Physical Review Letters, 95: 219802, 2005.
Haldeman, C. and Beggs, J.M. Critical branching captures activity in living neural networks and maximizes the number of metastable states. Physical Review Letters, 94: 058101, 2005.
Beggs, J.M., and Plenz D. Neuronal avalanches are diverse and precise activity patterns that are stable for many hours in cortical slice cultures. The Journal of Neuroscience, 24(22): 5216-29, 2004. https://www.jneurosci.org/content/24/22/5216.full
Beggs, J.M., and Plenz D. Neuronal avalanches in neocortical circuits. The Journal of Neuroscience, 23(35): 11167-77, 2003. http://www.jneurosci.org/content/23/35/11167.full
J. D. Johnson, D. Plenz, J. Beggs, W. Li, M. Mieier, N. Miltner, K. Owen. "Analysis of Spontaneous Activity in Cultured Brain Tissue Using the Discrete Wavelet Transform," p. 60, Third IEEE Symposium on BioInformatics and BioEngineering (BIBE03), 2003.
Beggs, J.M. A statistical theory of long-term potentiation and depression. Neural Computation. 13(1): 87-111, 2001.
Beggs, J.M., Moyer, J.R., McGann, J.P., and Brown, T.H. Prolonged synaptic integration in perirhinal cortical neurons. Journal of Neurophysiology. 83: 3294-3298, 2000.
Beggs, J.M., Chen, W. and Klukas, J. Network connectivity and neuronal dynamics. Chapter 3, pp. 91-116, in Handbook of Brain Connectivity (eds., Jirsa, V, and MacIntosh, R), Springer, 2007.
Beggs, J.M., Brown, T.H., Byrne, J.H., Crow, T.J., LeBar, K.S., LeDoux, J.E., and Thompson, R.F. Learning and Memory: Basic Mechanisms. In: Fundamental Neuroscience.(Eds: Floyd Bloom, Story Landis, James Roberts, Larry Squire, and Michael Zigmond), (Chapter 55, pp. 1411-1454), 1999.
Professor, Physics
biophysics
The physical sciences have had great success in describing how complex phenomena can emerge from the collective interactions of many similar units. Waves, turbulence, phase transitions, and self-organization are all examples of this.
Although the brain is tremendously complex, it is composed of many units, neurons, which appear to be similar. This resemblance has led many researchers to borrow concepts from physics in an effort to explain neural function. Indeed, many models predict that neural networks should exhibit metastable states like those seen in frustrated magnetic materials, and should operate near a critical point like that seen in matter at a phase transition. While this body of theory has prospered, experiments to test it have been few.
Recent advances in technology, however, have allowed thousands of interconnected neurons to be grown on microfabricated arrays of many electrodes. These "brains in a dish" can be kept alive for weeks while their spontaneous electrical activity is recorded. The large data sets produced by these experiments have allowed many of the hypotheses inspired by statistical physics to be examined in real neural tissue.
Our results indicate that living neural networks do in fact organize themselves so that many metastable states exist. In addition, these networks appear to operate at the critical point, producing distributions of event sizes that can be described by a power law. This surprising correspondence between biological data and physical theory may actually serve a purpose for the networks. Simulations indicate that metastable states can be used to store information, and that the critical point optimizes information transmission while preserving network stability. Future research combining biological experiments and computer simulations will be directed toward understanding fundamental emergent properties of living neural networks and how these properties may contribute to neural function.
Nir Friedman, Shinya Ito, Braden Brinkman, Masanori Shimono, R. Lee DeVille, Karin Dahmen, John M. Beggs, and Tom C. Butler. Universal critical dynamics in high resolution neuronal avalanche data. Physical Review Letters 108, 208102 (2012). https://journals.aps.org/prl/abstract/10.1103/PhysRevLett.108.208102
John M. Beggs and Nick Timme. Being critical of criticality in the brain. Frontiers in Fractal Physiology. (2012). http://www.ncbi.nlm.nih.gov/pmc/articles/PMC3369250/pdf/fphys-03-00163.pdf
Nick Timme, Wesley Alford, Benjamin Flecker, John M. Beggs. Towards resolving the multivariate information controversy. (2012). https://arxiv.org/abs/1111.6857
Benjamin Flecker, Wesley Alford, John M. Beggs, Paul Williams, and Randall Beer. Partial information decomposition as a spatiotemporal filter. Chaos 21, 037104 (2011). https://aip.scitation.org/doi/10.1063/1.3638449
Shinya Ito, Mike Hansen, Randy Heiland, Andrew Lumsdaine, Alan Litke, and John M. Beggs. Extending transfer entropy improves identification of effective connectivity in a spiking cortical network model. PLoS ONE 6(11): e27431. (2011) doi:10.1371/journal.pone.0027431. https://journals.plos.org/plosone/article?id=10.1371/journal.pone.0027431
Jon P. Hobbs, Jodi L. Smith, and John M. Beggs. Aberrant neuronal avalanches in cortical tissue removed from juvenile epilepsy patients. Journal of Clinical Neurophysiology. 2010 Dec. 27(6):380-6.
Wei Chen, Jon P. Hobbs, Aonan Tang, and John M. Beggs. A few strong connections: Optimizing information retention in neuronal avalanches. BMC Neuroscience. 2010 Jan 6;11(1):3. Open access at: https://bmcneurosci.biomedcentral.com/articles/10.1186/1471-2202-11-3
Fang-Chin Yeh, Aonan Tang, , Jon P. Hobbs, Pawel Hottowy, Wladek Dabrowski, Alexander Sher, Alan Litke, and John M. Beggs. Maximum entropy approaches to living neural networks. Entropy, 12: 89-106, 2010. Open access at: https://bmcneurosci.biomedcentral.com/articles/10.1186/1471-2202-11-3
Hsu, D., Chen, W., Hsu, M. and Beggs, J.M. An open hypothesis: is epilepsy learned, and can it be unlearned? Epilepsy and Behavior, Jun 21, 2008. (Epub ahead of print).
Tang, A., Jackson, D., Hobbs, J., Chen, W., Prieto, A., Smith, J.L., Patel, H. Sher, A., Litke, A and Beggs, J.M. A maximum entropy model applied to spatial and temporal correlations from cortical networks in vitro. The Journal of Neuroscience, 28(2): 505-518, 2008.
Beggs, J.M. The criticality hypothesis: how local cortical networks might optimize information processing. Philos Transact A Math Phys Eng Sci. 366(1864):329-343, 2008. https://royalsocietypublishing.org/doi/full/10.1098/rsta.2007.2092
Beggs, J.M. How to build a critical mind. Nature Physics 3(12): 834-835, 2007.
Hsu, D., Tang, A., Hsu, M., and Beggs, J.M. Simple spontaneously active Hebbian learning model: Homeostasis of activity and connectivity, and consequences for learning and epileptogenesis. Physical Review E 76: 041909, 2007.
Beggs, J.M. Neuronal Avalanche. Scholarpedia, p.6423, 2007. http://www.scholarpedia.org/article/Neuronal_avalanche
Hsu, D. and Beggs, J.M. Neuronal avalanches and criticality: A dynamical model for homeostasis. Neurocomputing, 69:1134-1136, 2006.
Beggs, J.M. and Haldeman, C. Beggs and Haldeman Reply: Physical Review Letters, 95: 219802, 2005.
Haldeman, C. and Beggs, J.M. Critical branching captures activity in living neural networks and maximizes the number of metastable states. Physical Review Letters, 94: 058101, 2005.
Beggs, J.M., and Plenz D. Neuronal avalanches are diverse and precise activity patterns that are stable for many hours in cortical slice cultures. The Journal of Neuroscience, 24(22): 5216-29, 2004. https://www.jneurosci.org/content/24/22/5216.full
Beggs, J.M., and Plenz D. Neuronal avalanches in neocortical circuits. The Journal of Neuroscience, 23(35): 11167-77, 2003. http://www.jneurosci.org/content/23/35/11167.full
J. D. Johnson, D. Plenz, J. Beggs, W. Li, M. Mieier, N. Miltner, K. Owen. "Analysis of Spontaneous Activity in Cultured Brain Tissue Using the Discrete Wavelet Transform," p. 60, Third IEEE Symposium on BioInformatics and BioEngineering (BIBE03), 2003.
Beggs, J.M. A statistical theory of long-term potentiation and depression. Neural Computation. 13(1): 87-111, 2001.
Beggs, J.M., Moyer, J.R., McGann, J.P., and Brown, T.H. Prolonged synaptic integration in perirhinal cortical neurons. Journal of Neurophysiology. 83: 3294-3298, 2000.
Beggs, J.M., Chen, W. and Klukas, J. Network connectivity and neuronal dynamics. Chapter 3, pp. 91-116, in Handbook of Brain Connectivity (eds., Jirsa, V, and MacIntosh, R), Springer, 2007.
Beggs, J.M., Brown, T.H., Byrne, J.H., Crow, T.J., LeBar, K.S., LeDoux, J.E., and Thompson, R.F. Learning and Memory: Basic Mechanisms. In: Fundamental Neuroscience.(Eds: Floyd Bloom, Story Landis, James Roberts, Larry Squire, and Michael Zigmond), (Chapter 55, pp. 1411-1454), 1999.